How To Say Lack Of Data
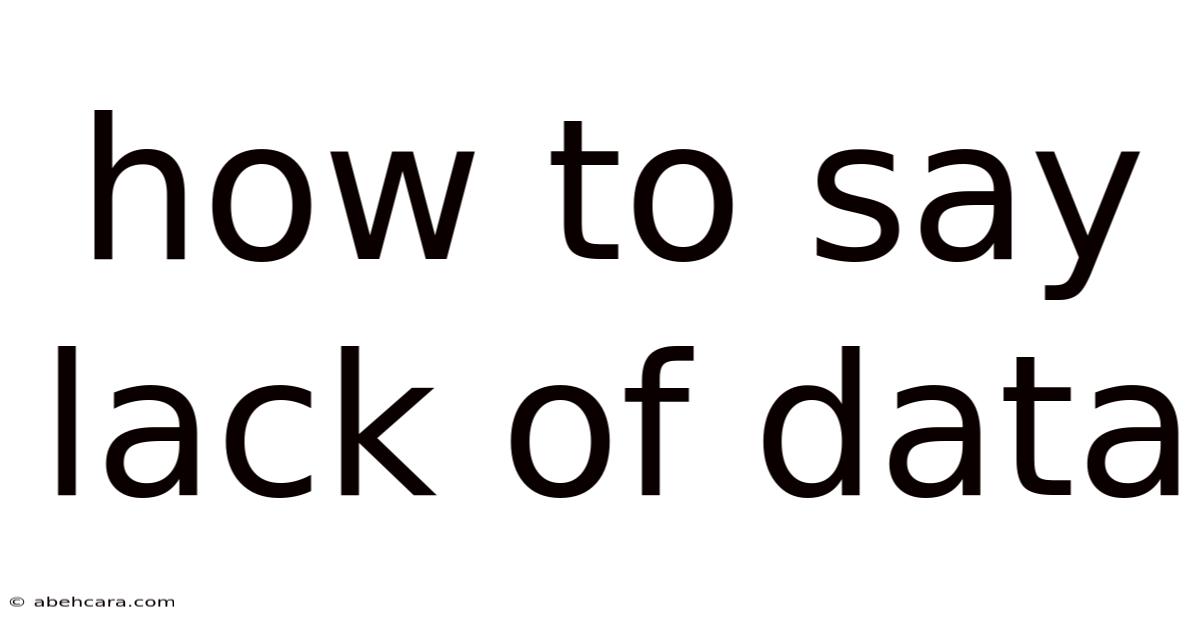
Discover more detailed and exciting information on our website. Click the link below to start your adventure: Visit Best Website meltwatermedia.ca. Don't miss out!
Table of Contents
How to Say "Lack of Data": Navigating the Nuances of Data Scarcity
Unlocking the Secrets of Data Scarcity: Key Insights and Discoveries
What challenges does inadequate data present in research and decision-making?
Data scarcity is a pervasive issue, significantly impacting the reliability and validity of analyses across diverse fields.
Editor’s Note: This article on navigating data scarcity was published today.
Data scarcity, or the lack of sufficient data to draw meaningful conclusions, is a pervasive challenge across numerous fields. From scientific research to business analytics, the absence of adequate data can severely hinder progress and decision-making. Understanding how to effectively communicate this data deficiency, both in writing and verbally, is crucial for maintaining transparency, managing expectations, and guiding future research or strategic planning. This article explores various ways to articulate the absence of data, providing context and highlighting the implications of this limitation.
This article explores the multifaceted nature of data scarcity, its impact on different disciplines, and strategies for effectively communicating the challenges it presents. Readers will gain a deeper understanding of how to accurately describe data limitations, mitigate their impact, and guide further data collection efforts.
This article draws upon a wide range of sources, including academic literature, industry reports, and best practices in data analysis and communication. A structured approach ensures that the insights are presented clearly and systematically, providing actionable guidance for researchers, analysts, and decision-makers facing the common hurdle of insufficient data.
Key Considerations when Describing Data Scarcity
Term | Description | Context |
---|---|---|
Data Sparsity | Data points are widely scattered, leading to unreliable estimations or predictions. | Spatial data, longitudinal studies, rare events |
Data Limitation | A general term referring to any constraint on the availability or quality of data. | Broad application across various contexts |
Insufficient Data | Simply stating that there isn't enough data to support a particular analysis or conclusion. | Straightforward and easily understood |
Data Gaps | Specific areas where data is missing or incomplete, often highlighting the nature of the missing information. | Crucial for identifying areas needing further data collection |
Limited Sample Size | Specifically addressing the small number of observations, potentially impacting statistical power and generalizability. | Statistical analysis, surveys, experiments |
Absence of Data | A more formal way to indicate the complete lack of relevant data for a particular analysis. | Academic research, scientific studies |
Unreliable Data | Data that is inconsistent, inaccurate, or prone to bias, rendering it unsuitable for analysis. | Focuses on the quality, rather than the quantity, of data |
Incomplete Dataset | A dataset that lacks essential variables or observations needed for a thorough analysis. | Emphasizes the incompleteness of the data itself |
Let's delve into the key aspects of effectively communicating a lack of data.
1. Defining the Scope of Data Scarcity
The first step involves clearly defining the extent of the data limitation. Is the data completely absent, or is there a partial dataset with significant gaps? Specify the type of data missing – are there insufficient quantitative measures, qualitative data, or both? For example, instead of simply stating "lack of data," consider phrases like:
- "The study was limited by the absence of longitudinal data on patient outcomes."
- "Insufficient quantitative data prevented a robust analysis of the market trends."
- "Significant gaps exist in the available demographic data, hindering a comprehensive understanding of the population."
2. Explaining the Implications of Data Scarcity
Once the nature of the data scarcity is clarified, explain its implications for the analysis or decision-making process. This involves discussing how the lack of data impacts the validity, reliability, and generalizability of findings. For instance:
- "The limited sample size may inflate the margin of error and reduce the statistical power of the analysis."
- "The absence of control group data limits our ability to establish causality."
- "Data gaps in the survey responses prevent us from drawing definitive conclusions about consumer preferences."
3. Suggesting Strategies for Mitigating Data Limitations
Proactively addressing the data scarcity by suggesting mitigation strategies demonstrates a proactive approach and enhances credibility. These strategies could include:
- "Further data collection is needed to validate these preliminary findings."
- "Future research could utilize alternative data sources to address the limitations of the current dataset."
- "A larger sample size would improve the precision and reliability of the analysis."
- "Employing imputation techniques may help to partially address missing data points." (Note that this should be used cautiously and with appropriate justification).
4. Using Appropriate Language and Tone
The language used to describe data scarcity should be precise, transparent, and appropriate for the intended audience. Avoid using vague or overly technical terms that may not be understood by non-experts. Maintain a neutral and objective tone, focusing on the facts rather than making excuses for the limitations.
Exploring the Connection Between Data Quality and Data Scarcity
Data quality and data scarcity are intertwined. Poor data quality can often exacerbate the impact of limited data. Inaccurate, inconsistent, or unreliable data, even if abundant, can lead to flawed conclusions. Addressing data quality issues is crucial, even when dealing with limited datasets. This involves:
- Data cleaning and validation: Identifying and correcting errors in existing data.
- Data verification: Ensuring the accuracy and consistency of data through multiple sources.
- Data transformation: Converting data into a suitable format for analysis.
Further Analysis of Data Quality Issues in the Context of Scarcity
The impact of poor data quality is amplified when data is scarce. A small number of erroneous data points can significantly skew results and lead to misleading conclusions. Therefore, a rigorous approach to data quality control is crucial when dealing with limited datasets. A structured approach can involve:
Stage | Actions | Example |
---|---|---|
Data Collection | Establish clear data collection protocols to minimize errors. | Implementing standardized questionnaires or using validated data collection tools. |
Data Cleaning | Identify and remove outliers, inconsistencies, and duplicate data points. | Using data cleaning software or manual inspection to identify and correct errors. |
Data Validation | Compare data from multiple sources to verify accuracy and consistency. | Cross-referencing data with official records or independent sources. |
Data Imputation | Filling in missing data points using appropriate statistical techniques. (Use cautiously) | Using mean imputation, regression imputation, or multiple imputation techniques. |
Frequently Asked Questions about Data Scarcity
-
How can I overcome data scarcity in my research? The best approach depends on the specific context. Consider alternative data sources, conduct more data collection, or refine your research question to focus on areas with available data.
-
What are the ethical implications of using limited data? Transparency is key. Clearly acknowledge data limitations and their potential impact on the conclusions. Avoid overgeneralizing or making claims that are not supported by the data.
-
How do I explain data scarcity to a non-technical audience? Use simple, clear language. Explain the implications of the missing data in terms that are easy to understand.
-
What statistical methods are suitable for analyzing data-sparse datasets? Consider Bayesian methods, bootstrapping, or techniques specifically designed for small sample sizes.
-
Can I still publish research with limited data? Yes, but you must clearly and transparently acknowledge the limitations and their implications. Focus on the robustness of your methodology and the insights you can still derive from the available data.
-
How can data visualization help in presenting findings with limited data? Visualizations can effectively highlight data patterns and emphasize uncertainties, communicating data scarcity in a transparent manner.
Practical Tips for Handling Data Scarcity
-
Define your research question carefully: Focus on a narrower scope to reduce data requirements.
-
Explore alternative data sources: Utilize publicly available datasets, government statistics, or industry reports.
-
Improve data collection methods: Use more efficient sampling techniques or increase the response rate in surveys.
-
Employ data imputation techniques carefully: Only use validated techniques and acknowledge the potential limitations.
-
Focus on qualitative data: Supplement quantitative data with richer qualitative insights through interviews or case studies.
-
Clearly communicate limitations: Transparency about data scarcity builds trust and credibility.
-
Conduct sensitivity analysis: Assess how the findings change when different assumptions are made about missing data.
-
Consider using data augmentation techniques: Generate synthetic data to supplement your existing data (use with caution and appropriate validation).
Final Conclusion
Navigating data scarcity requires a strategic and transparent approach. By understanding the nuances of describing data limitations, acknowledging the implications, and proactively suggesting mitigation strategies, researchers and analysts can build credibility and foster a more informed understanding of the challenges inherent in working with incomplete datasets. The key takeaway is not to shy away from acknowledging the limitations but to present them as opportunities for further exploration and refinement, guiding more robust research and decision-making in the future. Embrace transparency, and let the limitations of the data inform the narrative, leading to a more accurate and ethically sound representation of the findings.
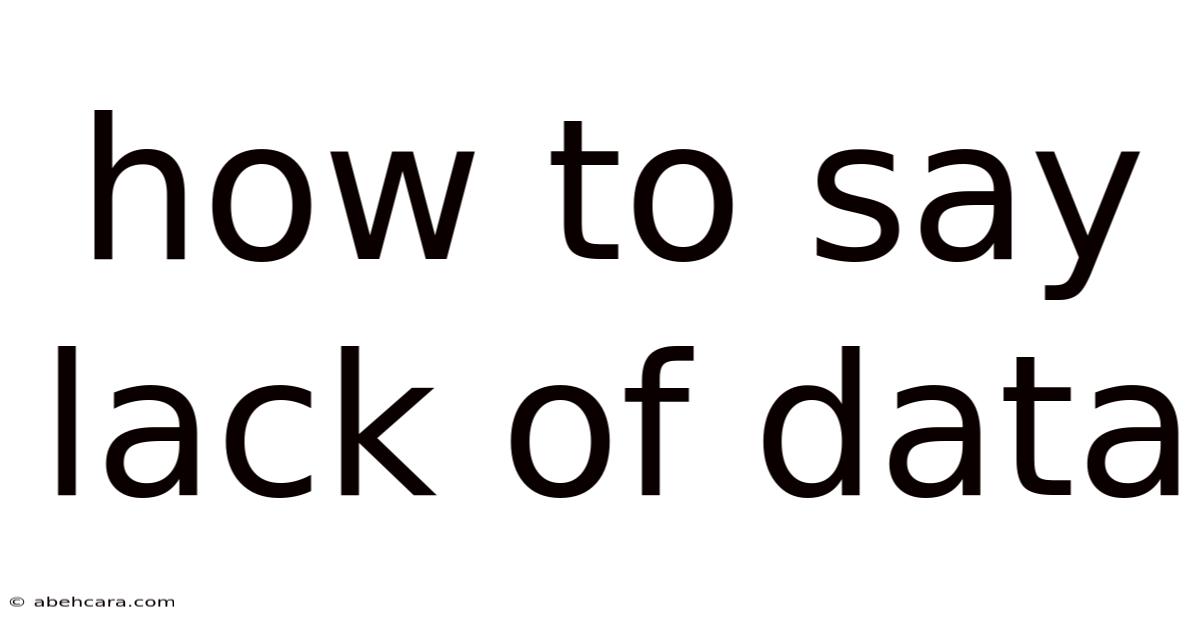
Thank you for visiting our website wich cover about How To Say Lack Of Data. We hope the information provided has been useful to you. Feel free to contact us if you have any questions or need further assistance. See you next time and dont miss to bookmark.
Also read the following articles
Article Title | Date |
---|---|
How To Say See You Tomorrow Formally | Apr 13, 2025 |
How To Say Son In Mexican | Apr 13, 2025 |
How To Say La In Korean | Apr 13, 2025 |
How To Say Thanks For Rakhi Gift | Apr 13, 2025 |
How To Say Sorry It S My Fault | Apr 13, 2025 |